Recently, Strava launched Athlete Intelligence, which uses AI to analyze your activities and provide personalized feedback. When I saw it, I couldn't help but reflect on my time with Addapp, the startup I co-founded almost a decade ago. At Addapp, we were doing something similar, but with the technology of that time.
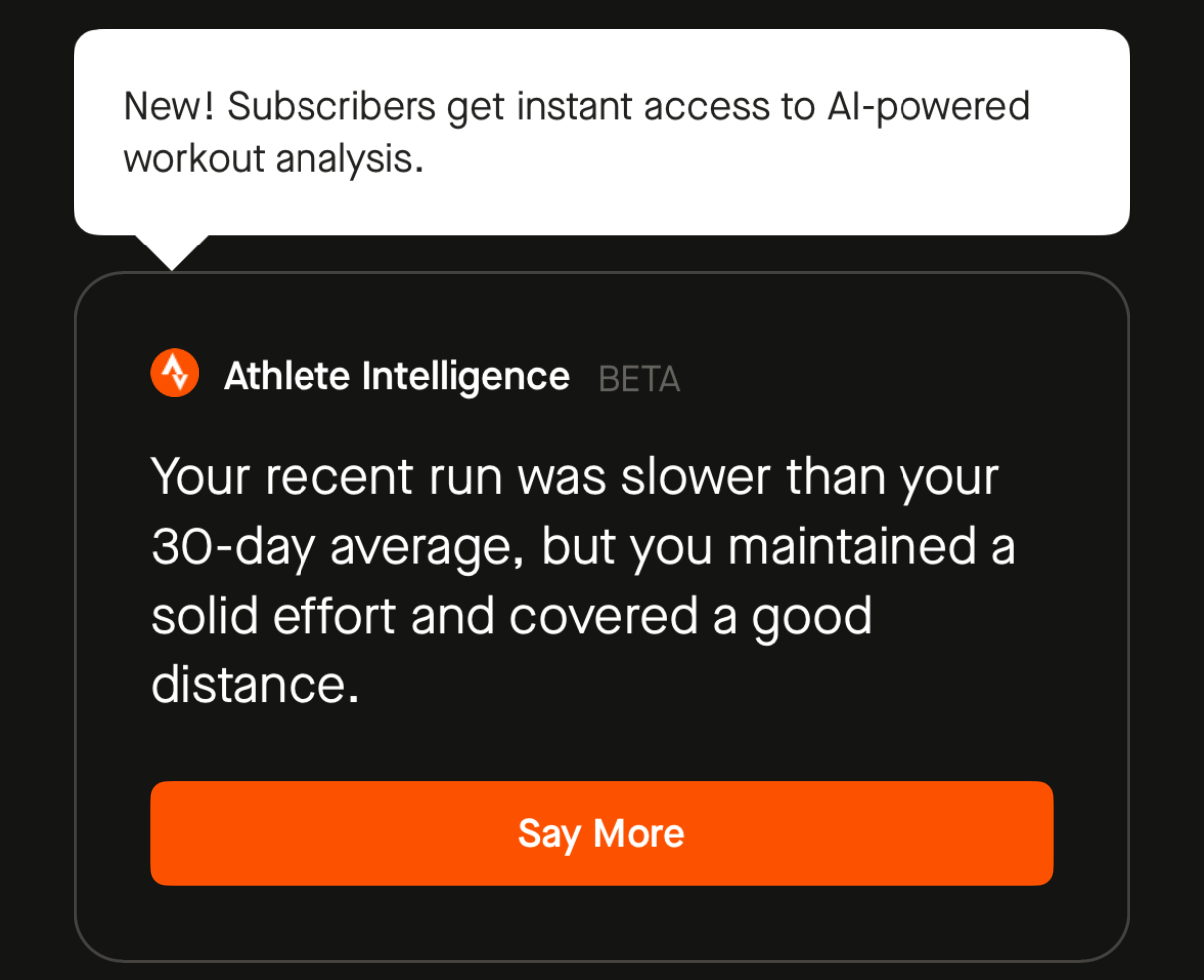
Addapp was ahead of time in gathering data from health-tracking providers like Apple Watch and Fitbit. Our goal was to transform this raw data into meaningful, unified insights. We could tell you if yesterday's run was faster or slower than usual and dig deeper into correlations, such as how your running might impact your sleep quality. It was fascinating work, and we were able to offer valuable insights with just traditional data science long before AI became the buzzword it is today.
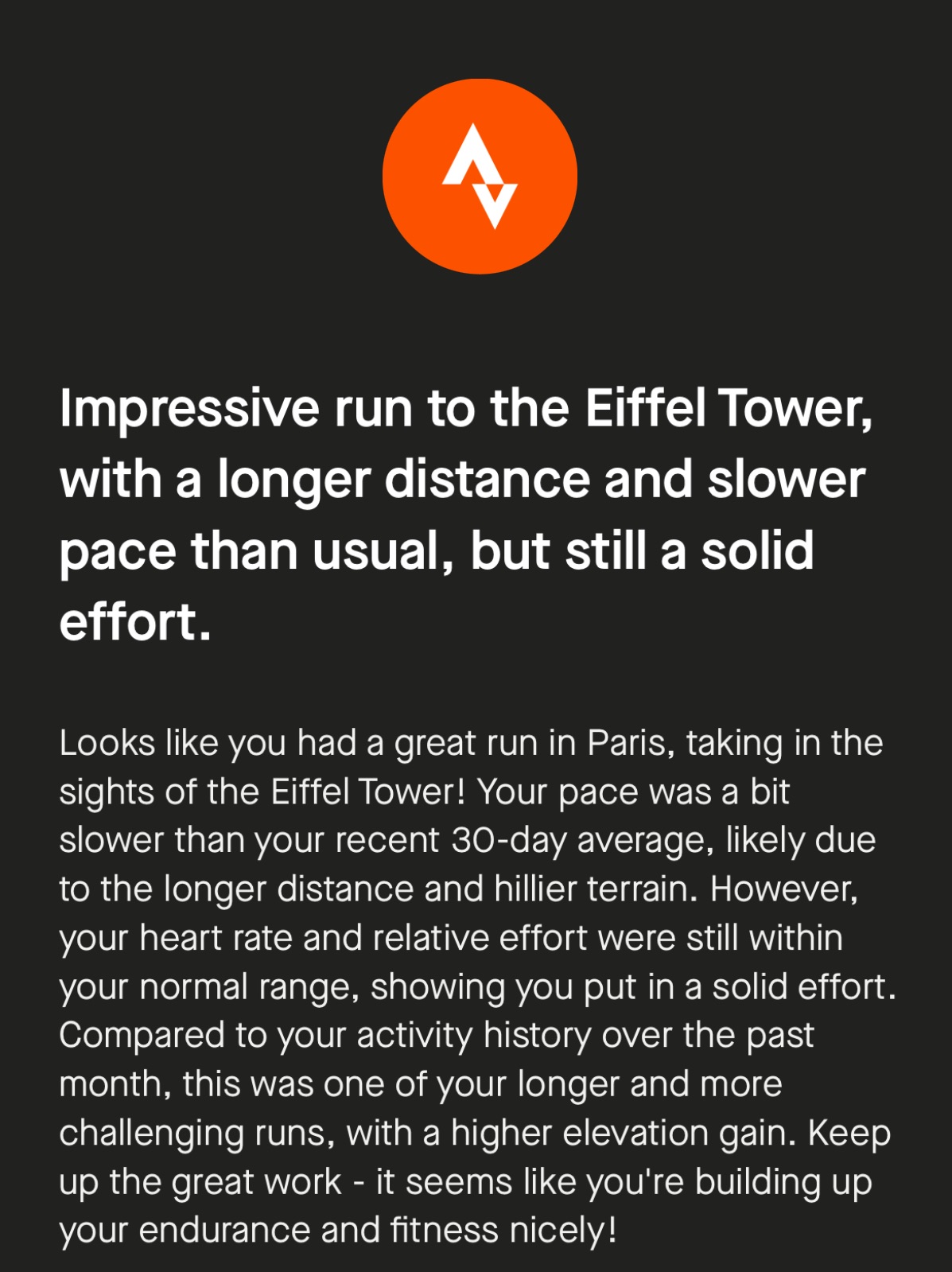
For example, two weeks ago, even before Strava announced the Athlete Intelligence publicly, I went for a training run in Paris. Strava later provided feedback, saying, "You did a great run to the Eiffel Tower, close to your longer-term average run in the last two weeks." While this is a nice acknowledgement, it's a very basic insight. At Addapp, we were way more advanced. Our system could correlate various data points to provide a deeper understanding, such as how a particular run might influence your sleep patterns or overall fitness trends.
Strava's Athlete Insights might look more polished now, especially with its natural language processing capabilities that give you a more conversational workout summary. But at its core, the concept remains the same—transforming raw data into something actionable and insightful. It's a familiar concept, and seeing how far the technology has come is exciting.
That said, the AI Strava now employs comes at a cost—both financially and in terms of complexity. At Addapp, we built our system in a lean, efficient manner. We didn't have to rely on expensive AI models. Instead, we used well-designed algorithms that could handle large datasets and deliver insightful results. It makes me wonder: Is today's AI-driven approach truly superior, or just a different means to the same end?
Looking back, it's also a reminder of how timing plays a massive role in the success of a product. Addapp may have been too early for mass adoption, while Strava is riding a wave where the market is ready and the technology is more accessible.
Ultimately, I'm proud of the work we did at Addapp. Seeing how ideas evolve, refine, and sometimes come back in new forms is fascinating. Watching how Strava and others advance in this space reinforces my belief that good ideas always find their way to the surface—sometimes, it's just a matter of timing.
Member discussion